A 'rule of 0.5' for the metabolite-likeness of approved pharmaceutical drugs
- PMID: 25750602
- PMCID: PMC4342520
- DOI: 10.1007/s11306-014-0733-z
A 'rule of 0.5' for the metabolite-likeness of approved pharmaceutical drugs
Abstract
We exploit the recent availability of a community reconstruction of the human metabolic network ('Recon2') to study how close in structural terms are marketed drugs to the nearest known metabolite(s) that Recon2 contains. While other encodings using different kinds of chemical fingerprints give greater differences, we find using the 166 Public MDL Molecular Access (MACCS) keys that 90 % of marketed drugs have a Tanimoto similarity of more than 0.5 to the (structurally) 'nearest' human metabolite. This suggests a 'rule of 0.5' mnemonic for assessing the metabolite-like properties that characterise successful, marketed drugs. Multiobjective clustering leads to a similar conclusion, while artificial (synthetic) structures are seen to be less human-metabolite-like. This 'rule of 0.5' may have considerable predictive value in chemical biology and drug discovery, and may represent a powerful filter for decision making processes.
Keywords: Cheminformatics; Drug-likeness; Genome-wide metabolic reconstruction; KNIME; Metabolite-likeness; Recon 2.
Figures
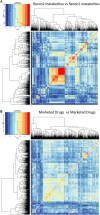
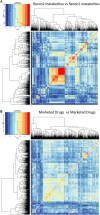
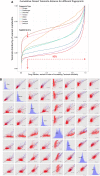
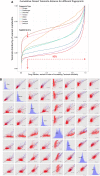
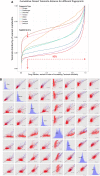
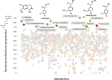
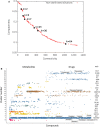
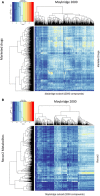
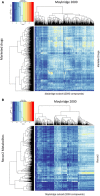
Similar articles
-
Drug repositioning for enzyme modulator based on human metabolite-likeness.BMC Bioinformatics. 2017 May 31;18(Suppl 7):226. doi: 10.1186/s12859-017-1637-5. BMC Bioinformatics. 2017. PMID: 28617219 Free PMC article.
-
Understanding the foundations of the structural similarities between marketed drugs and endogenous human metabolites.Front Pharmacol. 2015 May 13;6:105. doi: 10.3389/fphar.2015.00105. eCollection 2015. Front Pharmacol. 2015. PMID: 26029108 Free PMC article.
-
DrugMint: a webserver for predicting and designing of drug-like molecules.Biol Direct. 2013 Nov 5;8:28. doi: 10.1186/1745-6150-8-28. Biol Direct. 2013. PMID: 24188205 Free PMC article.
-
'Metabolite-likeness' as a criterion in the design and selection of pharmaceutical drug libraries.Drug Discov Today. 2009 Jan;14(1-2):31-40. doi: 10.1016/j.drudis.2008.10.011. Epub 2008 Dec 26. Drug Discov Today. 2009. PMID: 19049901 Review.
-
Drug discovery beyond the 'rule-of-five'.Curr Opin Biotechnol. 2007 Dec;18(6):478-88. doi: 10.1016/j.copbio.2007.10.005. Epub 2007 Nov 26. Curr Opin Biotechnol. 2007. PMID: 18035532 Review.
Cited by
-
MassGenie: A Transformer-Based Deep Learning Method for Identifying Small Molecules from Their Mass Spectra.Biomolecules. 2021 Nov 30;11(12):1793. doi: 10.3390/biom11121793. Biomolecules. 2021. PMID: 34944436 Free PMC article.
-
Drug repositioning for enzyme modulator based on human metabolite-likeness.BMC Bioinformatics. 2017 May 31;18(Suppl 7):226. doi: 10.1186/s12859-017-1637-5. BMC Bioinformatics. 2017. PMID: 28617219 Free PMC article.
-
Involvement of multiple influx and efflux transporters in the accumulation of cationic fluorescent dyes by Escherichia coli.BMC Microbiol. 2019 Aug 22;19(1):195. doi: 10.1186/s12866-019-1561-0. BMC Microbiol. 2019. PMID: 31438868 Free PMC article.
-
Relative Binding Free Energy between Chemically Distant Compounds Using a Bidirectional Nonequilibrium Approach.J Chem Theory Comput. 2022 Jun 14;18(6):4014-4026. doi: 10.1021/acs.jctc.2c00295. Epub 2022 Jun 1. J Chem Theory Comput. 2022. PMID: 35642423 Free PMC article.
-
The biology of ergothioneine, an antioxidant nutraceutical.Nutr Res Rev. 2020 Dec;33(2):190-217. doi: 10.1017/S0954422419000301. Epub 2020 Feb 13. Nutr Res Rev. 2020. PMID: 32051057 Free PMC article. Review.
References
-
- Bender A. How similar are those molecules after all? Use two descriptors and you will have three different answers. Expert Opinion on Drug Discovery. 2010;5:1141–1151. - PubMed
Grants and funding
LinkOut - more resources
Full Text Sources
Other Literature Sources